Some "Useful" A.I.
Mina Kimes (@minakimes.bsky.social), a sports journalist at ESPN, wrote on Bluesky this morning: "Every AI review is like: This isn't very useful and makes it harder to do simple tasks than if I just did it myself ..."
Every review of AI is like: This isn’t very useful and it makes it harder to do simple tasks than if I just did it myself. Riddled with errors. Can’t see anyone using this. I feel stupider having tried this. That said I believe it will soon make human cognition redundant and eradicate us all.
— Mina Kimes (@minakimes.bsky.social) February 6, 2025 at 7:17 AM
This attitude is prevalent. Many people don't (yet?) see a use-case or business model for generative-artificial intelligence or "Gen AI" aside from maybe the eradication of humanity. See, for instance the following Venn diagram from the same Bluesky thread.
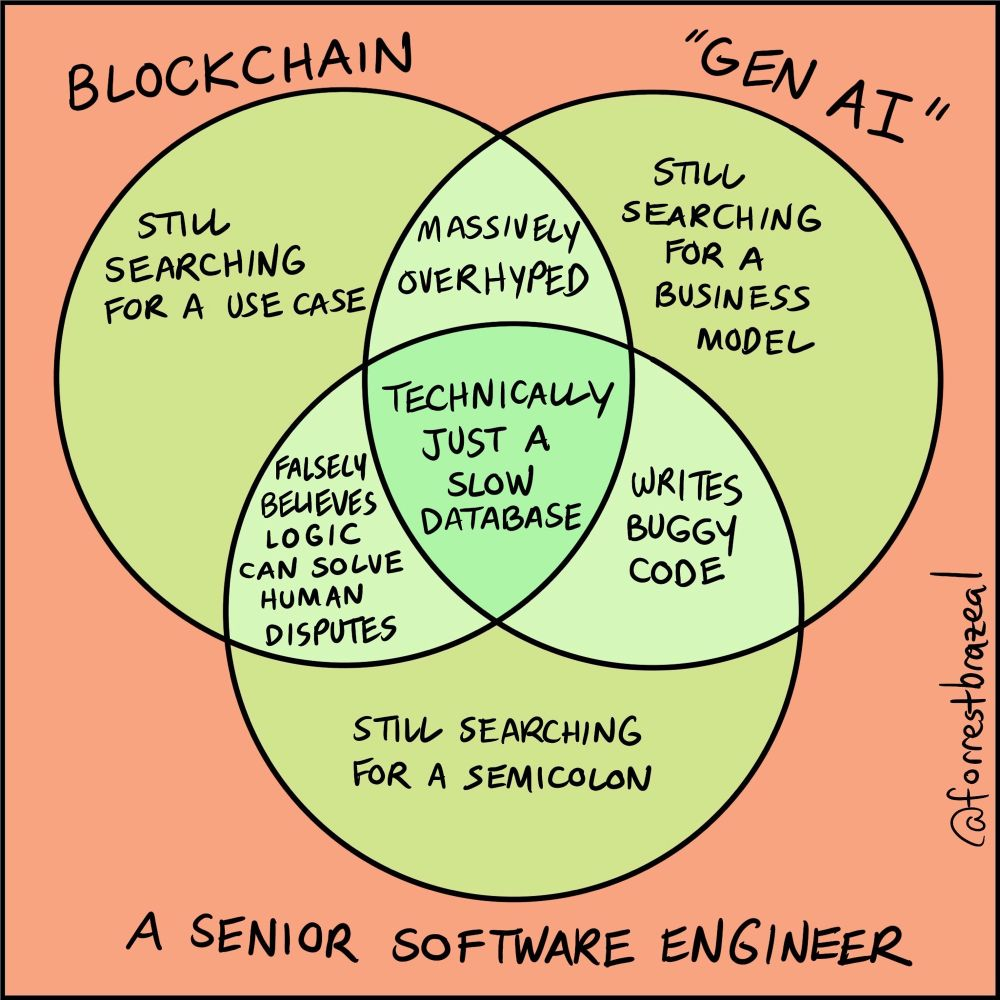
I do love a good Venn diagram!
Clearly, I and many others share this sentiment. It often feels like the services we get that involve "Gen AI" are intrusive, provide information that is not useful or is irrelevant to the questions we're asking, and ultimately, is a waste of time. At least that's a common perception.
There is another AI though that I want to spend a little time writing about today. Let's distinguish this "other" AI from "Gen AI" by just calling it "machine learning."
Machine learning was and is a term used in the data sciences that precedes the use of the term artificial intelligence in the same space. Science fiction writers and philosophers have been using the term artificial intelligence for many years, but the term has only recently been adopted to describe the work that data scientists do, at least in the public's perception of this work.
Machine learning, or artificial intelligence in the data sciences, uses a mathematical model and data to "train" a machine to make predictions about new data. In this sense, all AI is "Gen AI." A prediction from a model is what is being "generated."
We tend to call AI "generative" when the underlying model involves language, particularly, a Large Language Model (LLM). These models take language in the form of text or speech as their inputs. The predictions (i.e., the content that gets generated) are also in the form of language or speech meant to mimic that which a human would produce in reaction to similar inputs.
You can "prompt" ChatGPT with an idea for an essay or blog post, and ChatGPT will "write" the content that you asked for. You can enter a search query into just about any web browser, and an AI agent will seek to summarize content from your search. These results – whether from ChatGPT or your web browser – are not always accurate, or ethical uses for that matter, but aside from that, this describes the rough process behind the AI agent, the LLM, and the output it generates.
The "other" form of AI or machine learning involves non-language based inputs and outputs. This AI, along with "Gen AI" is making news as computing power continues to grow, and with it, more complex models.
One item in particular caught my eye this week.
On Monday, The Lancet published results from the "first randomised controlled trial investigating the use of AI in mammography screening." Instead of relying on just trained radiologists as screen readers to detect potential cancers from mammograms, the researchers trained a machine to assist in the screen-reading procedure.
The results were remarkable. "The AI-supported screen-reading procedure resulted in a significant increase in cancer detection ... without increasing the false-positive rate while reducing the screen-reading workload." Further, the increase in cancer detection was fairly consistent across all age groups 40 and up.
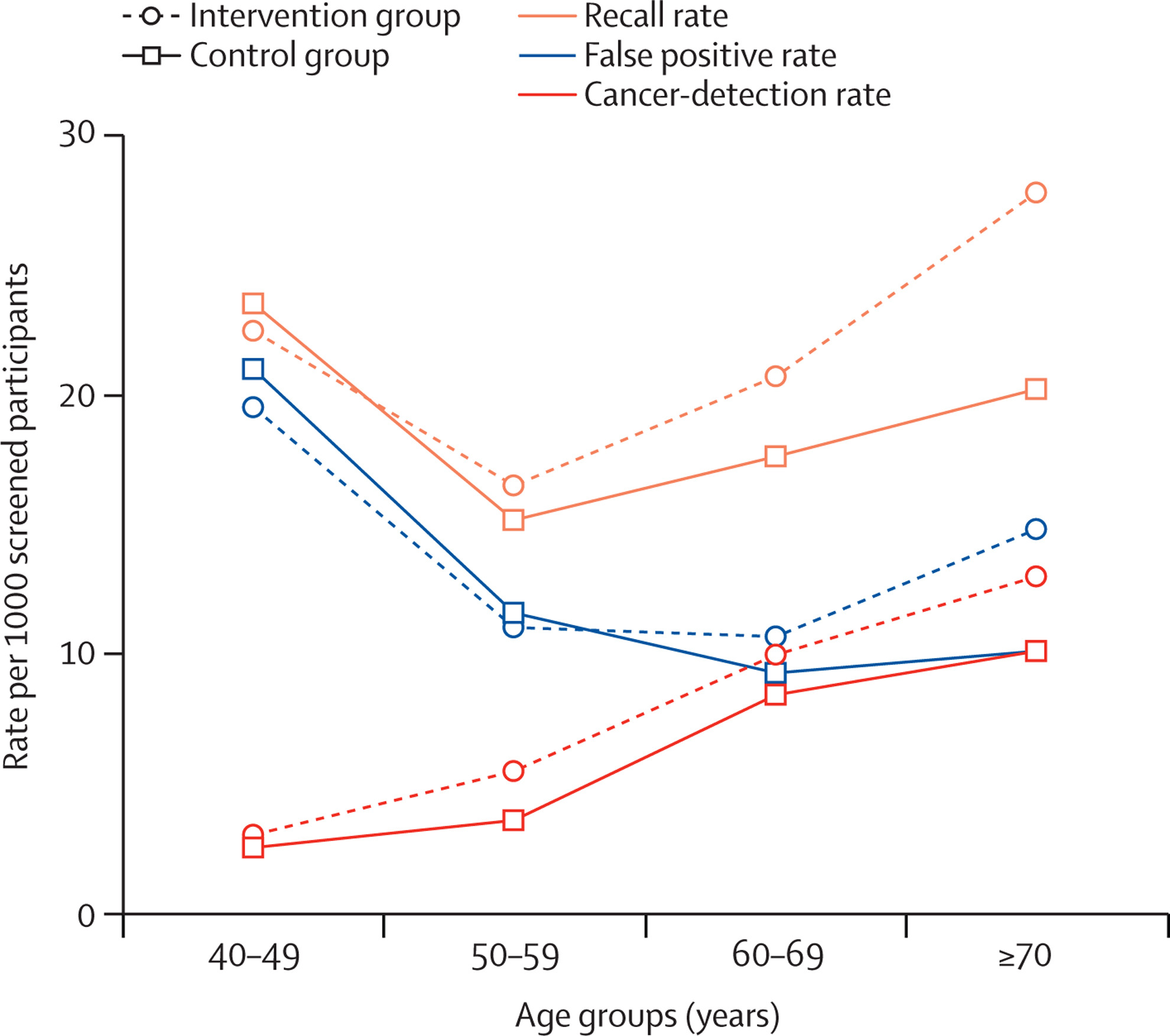
This type of application of artificial intelligence is perhaps more useful to society than what is being generated by LLMs. In this case, better detection of cancers from mammograms, particularly early-stage cancers, could literally save women's lives.
LLMs play a role in the medical community too. A chatbot on WebMD uses an LLM in the same way that a chatbot on an airline's website does to help you change your plane reservation. Maybe not very well, but it's often a start in a longer process.
But, the use of non-language-based AI, such as that described in The Lancet article, to conduct primary research that ultimately improves outcomes and lessens the workloads of physicians, seems to me to be a better use of the technology.
This form of AI gets a little less press than "Gen AI" – I'll try to do my part to bring attention to some of these as I come across them. It is critically important though that we stay tuned-in to findings from non-language based AI and not just cast all AI into the category of making it "more difficult to do simple tasks."
That's not at all a swipe at Ms. Kimes, who is expressing a commonly-held perception about "Gen AI" that I happen to very much agree with (maybe not the human eradication part, but who knows at this point?). It is merely an exhortation to be aware of some applications of AI that we might not have heard about in other places.
Stay tuned.
Member discussion