Why is Gen AI Still So 'Sloppy'?
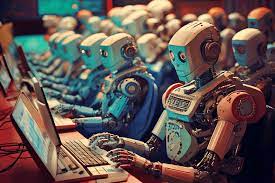
There is a new-ish term being used to describe a lot of generated AI content: "AI slop" or sometimes simply "slop." Slop in this context usually refers to AI-generated images that appear to be absurd or unrealistic, and is frequently used to farm clicks on social media.
DALL-E 2, an image-generating model from OpenAI introduced in 2022, is being used to create more than 2 million images per day. That is just from one program.
These images, especially those that include humans, often have indications that they are artificially-generated. Extra fingers and extra limbs are common distortions, along with odd facial asymmetries, and eyes that seem vaguely off somehow. In images that might not include humans, misaligned shadows and lighting that makes the image seem almost too perfect or "Disney"-fied can be indications that an image might be AI-generated. Distorted text on signs, billboards, and branded products are still another telltale indicator.
These "sloppy" AI-generated images fit squarely into the "uncanny valley," or the feeling of unease we feel when an image or object approaches that of a human likeness, but not quite. When a human is depicted as a character in a cartoon or comic strip such as Charlie Brown in Peanuts or Bart Simpson in The Simpsons, we easily recognize it for the caricature that it is. As images and other objects depicting humans become more and more realistic, we attribute them more human qualities. When we look at an actual photograph of a human, we recognize it as such and attribute more human qualities to the photo than we would to a caricature. However, there's a point where a depiction is almost there, but not quite. Something is just "off" about it. This is the "valley" of perception or affect, where the depiction itself will generate a feeling of eeriness or uncanniness in the observer.
AI image generators are getting better at avoiding the uncanny valley. A generated image was used last fall to criticize President Biden's response to Hurricane Helene. The image depicted a young girl holding a puppy fleeing the aftermath of the hurricane. While the image had many indications of being "fake," it still managed to fool a lot of people into believing the intended message.
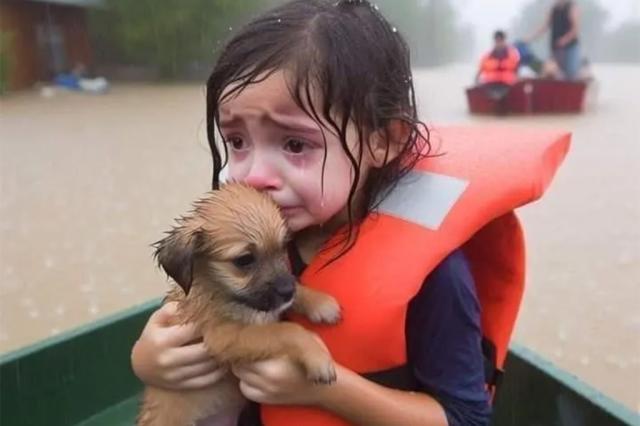
Scammers are also using AI-generated content to dupe people out of their savings. In a well-publicized instance, a French woman was convinced to divorce her husband and transfer $850,000 to scammers posing as Brad Pitt by sending her what appeared to be selfies and messages from the actor.
Even with all this hyper-realism, there is still a lot of slop in AI-generated images. Last week, after naming himself chair of the Kennedy Center, President Trump posted an image of himself conducting an orchestra. The image was easily-recognizable as having been AI-generated in the distortions of his fingers and face and from the unrealistic lighting and shadows.
Why are these images still so sloppy?
The answer to that question is that these images are being generated based on statistical patterns without a complete understanding of the real world. The statistical patterns are drawn from a large group of training data – real images in this case. If fingers accompany other fingers or teeth in a toothy grin accompany other teeth, an AI image generator may try to cram in too many fingers or teeth within what it considers reasonable space limitations. Effectively, the image-generating software is trying to match statistical patterns by placing like components together, and will frequently make what we consider to be "mistakes."
This tendency extends from text generated from large language models (LLMs) in programs such as ChatGPT (another OpenAI product), Claude, and Google LaMDA.
These models make a statistical "judgement" based on their training data of what words should accompany other words. This can be an effective way to regurgitate information, but like in image generation, it often lacks in real-world common knowledge.
A good example is Google LaMDAs – integrated into the Chrome browser – response to the question of "does water freeze at 27 degrees fahrenheit?"
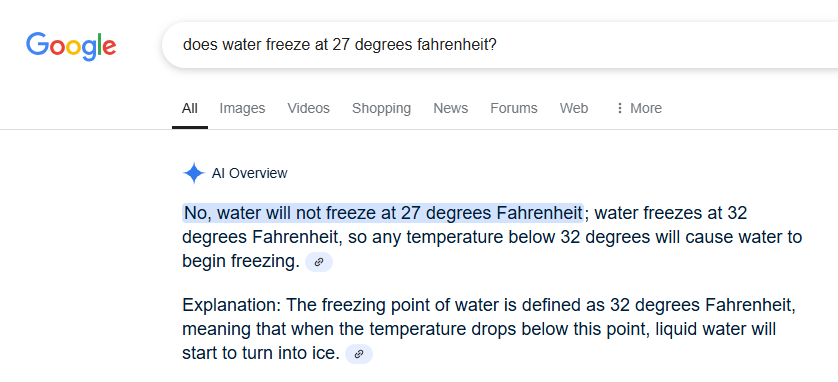
The second clause of the AI-generated summary gets the general principle exactly correct. Since under most conditions, water freezes at 32 degrees Fahrenheit, "any temperature below 32 degrees will cause water to begin freezing."
The first clause though, responding directly to the specific query, gets things backwards. Since 27 degrees is not 32 degrees – the point where Google LaMDA has been trained that water will begin freezing – the model tells us that water will not freeze at 27 degrees. This appears to contradict the second statement, but statistically, the two statements are in perfect agreement. The LLM after all is not a mathematical processor. It has no way of knowing that "27 degrees is below 32 degrees." It only knows that "27 degrees is not 32 degrees." In other words, the model has no reasoning abilities.
Because these models can only respond to statistical patterns present in language, they are – at least as of yet – unable to understand a story, let alone write one. They can "read" a novel, and repeat back key elements of it, but they cannot form an understanding of it. It can tell us "whodunit" at the end of a murder mystery, but it can't generate the logic or the coherent story needed to bring us to that point.
That's why AI-generated content can be self-contradictory, as in the above example, and many times, flat out wrong. This "feature" destroys Google's credibility. We humans are reasoning creatures, and immediately recognize when one piece of information contradicts another, especially when those are presented side-by-side. At minimum, these contradictions should cause cognitive dissonance, a phenomenon that comes into play in the "uncanny valley" too.
The LLM, having no reasoning ability, also has no ability to sense cognitive dissonance. Again, it's just repeating information based on statistical patterns. In this vein, these AI models are great for activities like code completion, saving time and resources for repetitive tasks, but they are less fine-tuned for any task that requires more thought.
From a brand/value perception, Google would be better off just returning websites that respond to our queries – as it has done since its invention in 1998 – rather than returning AI slop, which has a strong potential to be self-contradictory.
This is completely separate from the large amount of associated computing resources and costs – in infrastructure and electricity – that must be expended to make the models run.
Google, OpenAI, and other companies creating these AI generators are well aware of these flaws. Their reaction has often been that it's a new technology, and like any new technology, we need to be patient as the kinks are worked out. In effect, they're apologizing, in advance, for the flaws in their products, while at the same time encouraging people to use them. What a weird advertising strategy!
ChatGPT did just that in their Super Bowl commercial last week. They spent $14 million on a 60-second ad comparing ChatGPT to other technological breakthroughs, and claiming that it represents "the dawn of a new era," warts an all. The ad conveys the message that other technologies were created in fits and starts and that that's true of GenAI as well.
I don't remember the crypto industry advertising along similar lines in the Super Bowl three years ago. I only remember the humor and unbridled enthusiasm – though a lot of that has faded now.
It feels like Gen AI is becoming integral to everything we do when we're online. There is reason to be very cautious with the images and text it generates, and to know the reasons why.
Member discussion